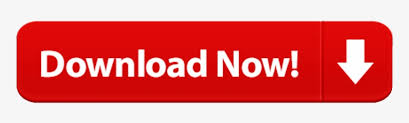
First we’ll look at a log-transformed dependent variable.

We’ll keep it simple with one independent variable and normally distributed errors. To get a better understanding, let’s use R to simulate some data that will require log-transformations for a correct analysis.
#Interpret ford as built data how to#
It’s nice to know how to correctly interpret coefficients for log-transformed data, but it’s important to know what exactly your model is implying when it includes log-transformed data. What Log Transformations Really Mean for your Models Example: For every 20% increase in the independent variable, our dependent variable increases by about (1.20 0.198 – 1) * 100 = 3.7 percent. From this specification, the average effect of Age on Income, controlling for Gender should be. 80, while for Females, Income and Age have a correlation of r. For x percent increase, calculate 1.x to the power of the coefficient, subtract 1, and multiply by 100. To illustrate, I am going to create a fake dataset with variables Income, Age, and Gender.My specification is that for Males, Income and Age have a correlation of r. For every 1% increase in the independent variable, our dependent variable increases by about 0.20%. Interpret the coefficient as the percent increase in the dependent variable for every 1% increase in the independent variable. Both dependent/response variable and independent/predictor variable(s) are log-transformed.Example: For every 10% increase in the independent variable, our dependent variable increases by about 0.198 * log(1.10) = 0.02. For x percent increase, multiply the coefficient by log(1.x). For every 1% increase in the independent variable, our dependent variable increases by about 0.002.
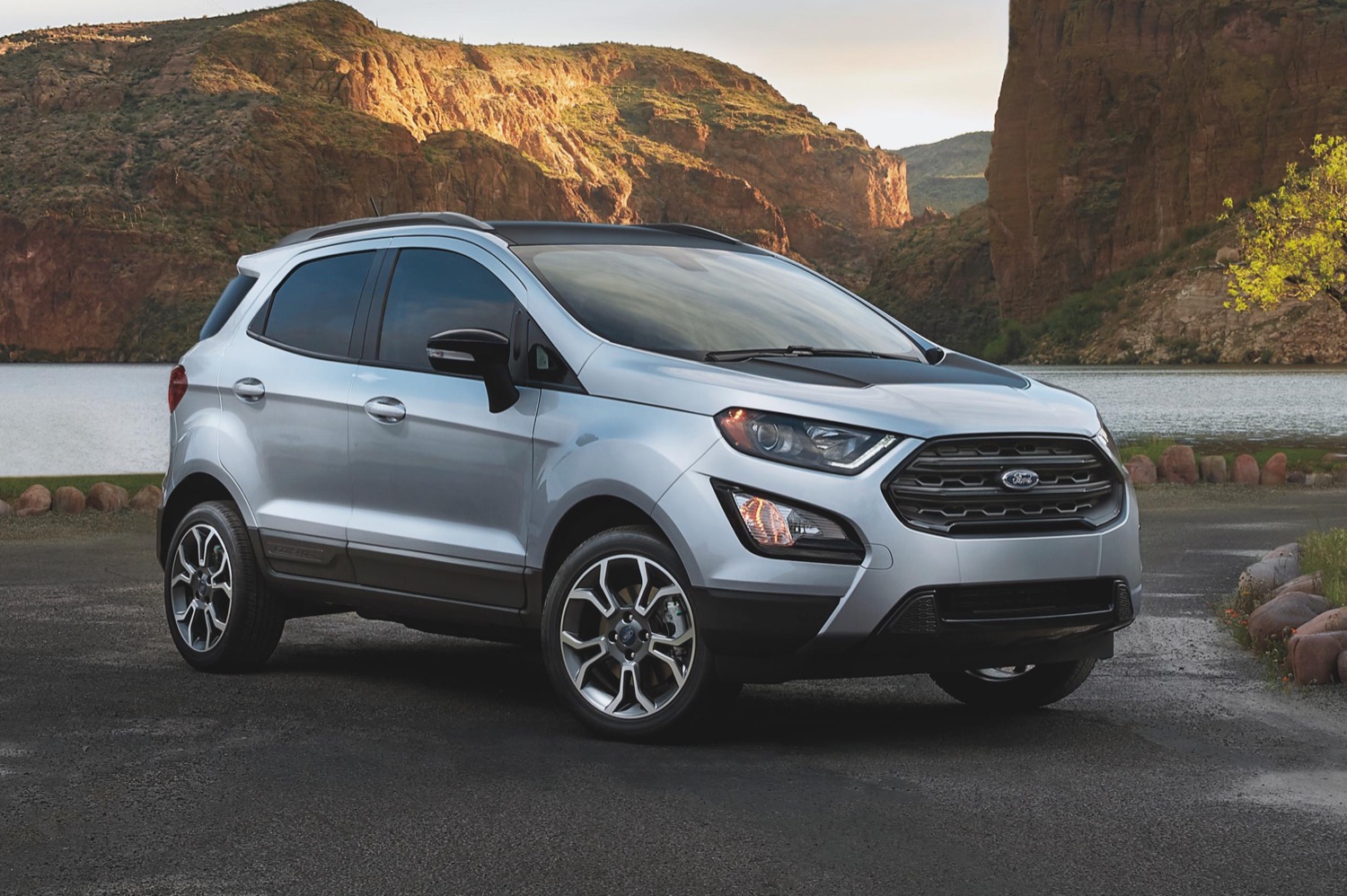
This tells us that a 1% increase in the independent variable increases (or decreases) the dependent variable by (coefficient/100) units.
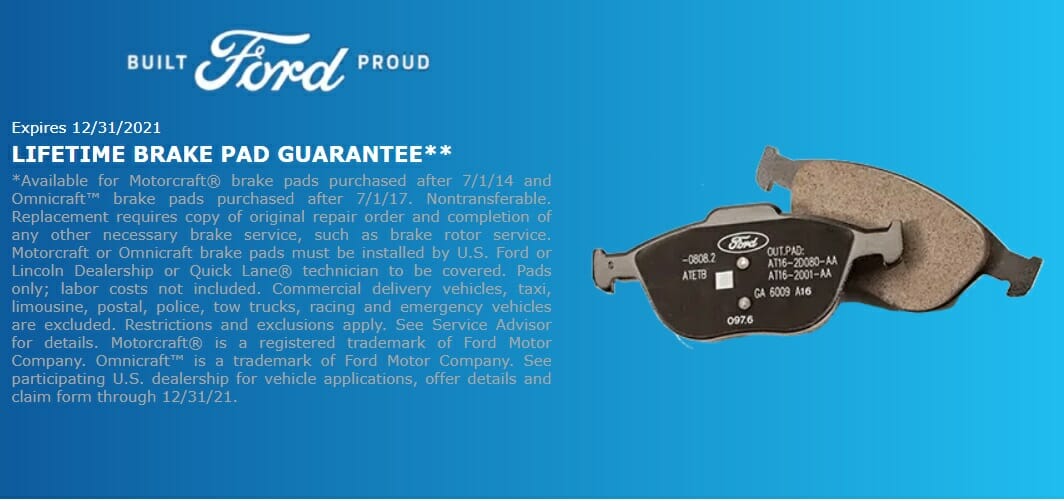
Let’s say we fit a linear model with a log-transformed dependent variable. But while it’s easy to implement a log transformation, it can complicate interpretation. Yet another is to help make a non-linear relationship more linear. Another reason is to help meet the assumption of constant variance in the context of linear modeling. If we’re performing a statistical analysis that assumes normality, a log transformation might help us meet this assumption. Why do this? One reason is to make data more “normal”, or symmetric.
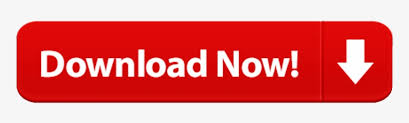